AI models ‘secretly’ learn long before they show their skills, researchers have found
1 month ago Benito Santiago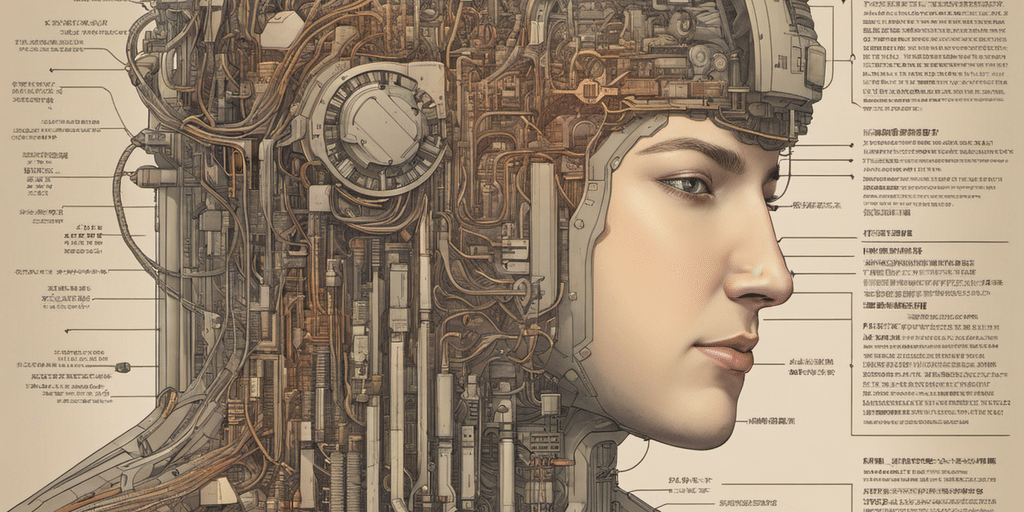
Modern AI models have latent abilities that emerge spontaneously and consistently during training, but these abilities remain hidden until they are prompted in specific ways, new research from Harvard and the University of Michigan shows.
The study, which analyzed how AI systems learn concepts like color and size, found that models often mastered these skills earlier than standard tests—a finding that has major implications for AI safety and development.
“Our results show that measuring the capabilities of an AI system is more complex than previously thought,” the research paper said. “A model may appear to be incompetent when given formal questions, while having advanced abilities that only come out in certain situations.”
This development joins a growing body of research aimed at identifying how AI models develop capabilities.
Anthropologists have unveiled a “learning dictionary” that mapped millions of neural connections in Claude's language model to specific concepts that AI understands, Decrypt reported earlier this year.
Although the approaches differ, these studies share a common goal: to bring clarity to what is essentially the “black box” learning process of AI.
“We discovered millions of features that correspond to concepts ranging from concrete objects such as people, countries and famous buildings to abstract ideas such as emotions, writing styles and reasoning steps,” Anthropic said in the research paper.
The researchers conducted extensive experiments using diffusion models – the most popular architecture for generative AI. Tracking how these models learned to process basic concepts, they found a consistent pattern: Skills emerged at different stages, marked by sharp transition points as the model acquired new skills.
Models have demonstrated conceptual ability up to 2,000 levels of training ahead of what can be distinguished from a standard test. Strong concepts appeared around 6,000 levels, while weak ones appeared around 20,000 levels.
When researchers adjust the “concept signal”, the clarity of the ideas presented in the training data.
They found direct correlations with learning speed. Alternative induction methods can reliably bring out latent abilities long before they appear in standard tests.
This “hidden emergence” phenomenon has significant implications for AI security and evaluation. Traditional benchmarks can dramatically overestimate what models can do, which can be both useful and empowering.
Perhaps most surprisingly, the team found several ways to access these hidden abilities. Using techniques they call “linear latent interpolation” and “oversampling,” researchers can reliably extract sophisticated features from models long before these capabilities are observed in standard experiments.
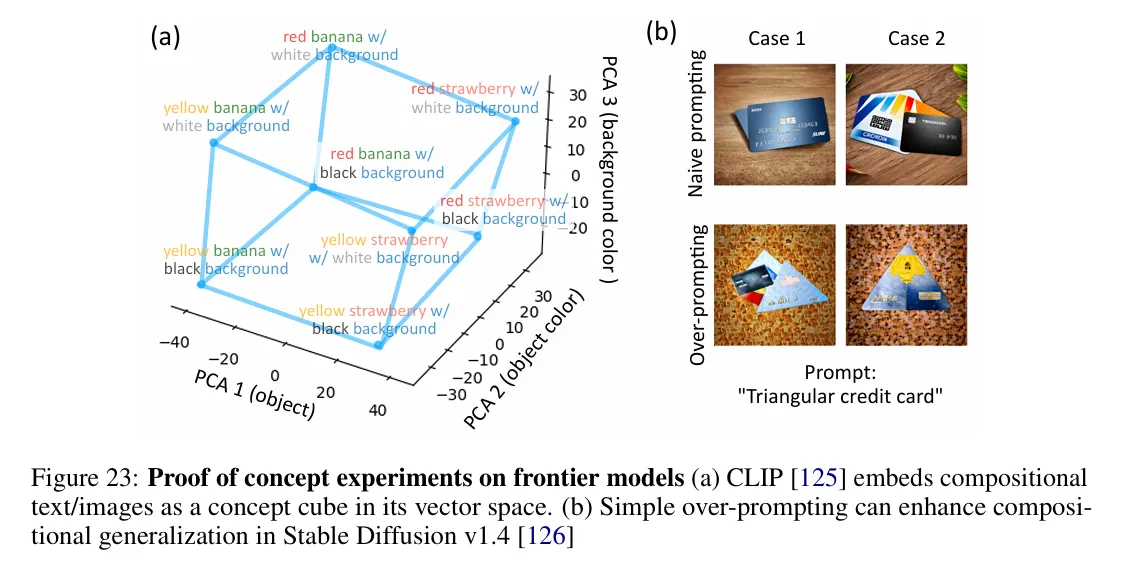
In another case, researchers learned to use complex features such as gender presentation and facial expressions before AI models could reliably demonstrate these skills with standard prompts.
For example, models can correctly generate “smiling women” or “men with hats” independently before combining these features – but detailed analysis shows that they mastered the combination too early. They simply couldn't explain it with normal inspiration.
The sudden emergence of abilities observed in this study may at first seem similar to a stroke—where models suddenly show perfect test performance after extended training—but there are key differences.
While entrainment occurs after a training field and involves the gradual improvement of representations over the same distribution of information, this study demonstrates skills that emerge during active learning and generalize outside of the distribution.
The authors found sharp transitions in the model's ability to manipulate concepts in new ways, suggesting distinct phase changes rather than process representation improvements seen in turbulence.
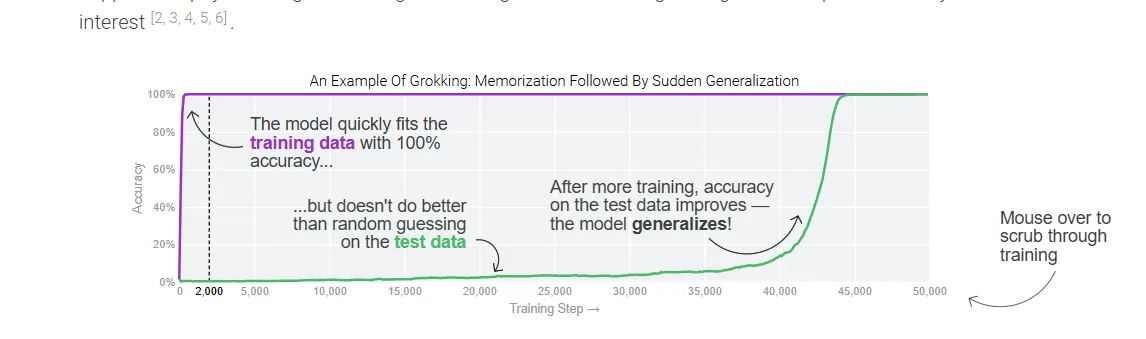
In other words, AI models seem to internalize concepts much better than we thought, failing to demonstrate their skills—how some people can understand a movie in a foreign language but still struggle to speak it correctly.
For the AI industry, this is a double-edged sword. The existence of hidden capabilities shows that models can be more powerful than previously thought. Still, it proves how difficult it is To fully understand and control what you can do.
Companies developing large language models and image generators may need to improve their testing protocols.
Traditional criteria, while still valuable, may need to be supplemented by more sophisticated assessment methods that can identify hidden abilities.
Edited by Sebastian Sinclair.
Generally intelligent newspaper
A weekly AI journey narrated by a generative AI model.