Big tech companies like IBM and Amazon are leading the AI boom with new tools
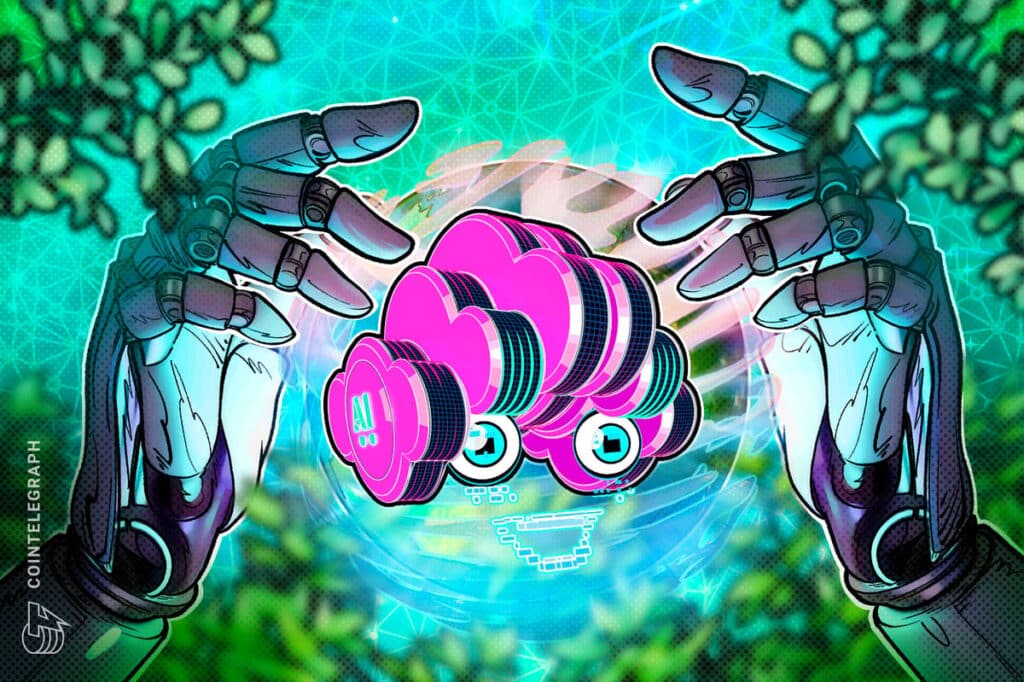
Over the past year or so, generative artificial intelligence (AI) has gained a lot of attention in the global technology landscape.
This is largely due to the new ability to transform how businesses and individuals approach problem solving, creativity and decision making. In fact, the versatility and efficiency of generative AI applications have led to their adoption in various industries, from healthcare to entertainment, as evidenced by the rapidly increasing market size.
As of 2023, the global artificial intelligence market is valued at $12.1 billion. However, some projections show that this figure could rise to $119.7 billion by 2032.
In addition, in 2022, when discussions around this technology are not yet mainstream, early AI startups managed to raise $2.6 billion in 110 deals, reaching $50 billion by 2023, such as OpenAI, Anthropic, and well-known companies. Inflection AI is due to earn several billion dollars each.
Another clear indication of increased interest in this space is the increasing number of searches related to the term “generative AI.” As you can see from the chart below, demand for OpenAI's ChatGPT platform has skyrocketed since its release — peaking in June — especially in countries like Singapore, China, Hong Kong, India, and Israel.
Thus, as the realm of AI-enabled technology continues to evolve, so does the scope of its applications, leading more companies to integrate these technologies into their operations.
Ilan Rachmanov, founder and CEO of ChainGPT.org – a provider of AI infrastructure for blockchain entities and Web3 projects – told Cointelegraph that “most popular products can now engage with generative AI and use it as a competitive edge. Also, we know what generative AI is capable of, but we still have limited understanding of how it will change in the long term as more and more organizations and individuals adopt the technology and train more and more models on relevance. Datasets.”
The main components of exploring generative AI
At the start of the new year, JPMorgan announced the release of DocLLM, a large language model (LLM) dedicated to multimodal document understanding. It can analyze and process information related to a variety of corporate documents – from forms and invoices to contracts and reports – often containing complex combinations of text and layout.
What sets DocLLM apart is its unique operational design, which avoids the heavy reliance on image encoders among existing multimodal language models. Instead, it focuses on bounding box information, integrating spatial structures more effectively. This is achieved through a novel split-space focusing mechanism that improves the focusing process in traditional transformers.
Amazon has upped its generative AI game by integrating a new tool to help sellers on its platform. It generates accurate and engaging product descriptions, greatly simplifying the process of listing new products. It is popular among most Amazon sellers.
Latest: US Elections: Are CBCCs Becoming ‘Hypo-Politicized'?
Mistral is the new expert hybrid or SMoE model in the developer community thanks to its speed, efficiency and extensive features. The model is based on open source, which makes it possible for devs to create special language models with limited resources.
DeepMind, a subsidiary of Google, continues to be a significant player in the generative AI arena. Their growth is evident in services like Google Brain and Google Translate. A recent contribution is the launch of Bard AI, a chat bot that mirrors the capabilities of Chat GPT and allows users to generate high-quality text and creative content.
Amazon Web Services (AWS) has made its mark by introducing Bedrock, a service that provides access to various models from different AI companies. Bedrock is well known for its comprehensive set of developer tools that help build and scale generative AI applications.
Cloud-based software company Salesforce has launched its customer relationship management platform—collectively referred to as “Insight GPT”—that maximizes unified customer engagement and personalization.
Finally, IBM released its Watson AI platform, which combines generative AI techniques with natural language processing (NLP) and machine learning (ML).
What does the future hold for generative AI?
Although the future of generative AI looks poised for transformational growth, the field is still treading uncharted ground full of promise and challenges. According to Rakhmonov, the direction of AI-driven technologies still largely depends on the development of models that are not only reliable, but also bring tangible value to their users:
“The future of generative AI is somewhat uncertain as it evolves with wider adoption and more data. However, the ‘black box' nature of many AI models poses a significant challenge as it can make it difficult to verify the reliability of data and insights. Lack of clarity about how AI models will produce results is a major challenge for mainstream AI. Public support may decline.
Similarly, Scott Dykstra, chief technical officer and co-founder of Space & Time — an AI-enabled, Microsoft-backed decentralized data store — told Cointelegraph that despite much hype around generative AI, the truth is there. The issue is more complicated.
As things stand, Dykstra said, most Fortune 500 companies are moving conservatively in the generative AI space, indicating that most are happy to “simply add AI Chabot to their website and call it a day.” Then he continued:
“The problem is that enterprises need to move at enterprise scale, and it's too expensive to do that today. While GPT-4 is a clear leader in productivity quality, it is also cost-effective for enterprise production-grade workloads. Across the board, as token prices fall, we should see more tools for faster valuation and more generation of retrieval.
Problems hindering the development of generative AI
As mentioned earlier, the evolution of generative AI is not a barrier. Dykstra believes that a critical technical challenge for generative models (such as LLMs) will be the speed of their respective simulation streams. “What we want for a truly LLM-oriented Internet is sub-second scanning speed, which is incredibly challenging,” he added.
On the development front, Dykstra believes that while progress has been made with AI-driven coding tools, a breakthrough in “no-code” solutions is yet to be seen. A no-code solution is a software development approach that requires few programming skills to quickly build an application.
“Many projects are using GPT-4 to code in large codebases, but the no-code design remains unaddressed due to the complexity of contextualizing the entire codebase,” he said.
Latest: Crypto is full of fake scams, don't let them steal your money
Rachmanov, on the other hand, focused on the broader landscape of impact on generative AI. He believes that regulatory measures from leading governments will be a key factor to monitor when defining acceptable AI practices.
What's more, he believes we could be in a global race for AI dominance, especially between major tech players and countries like the United States and China.
“Among the critical conversations shaping the future of AI are computing power and chip manufacturing,” he said.
So, as we move forward with technologies like AI, ML and NLP, it will be interesting to see how the global digital landscape will evolve and grow over the next decade.