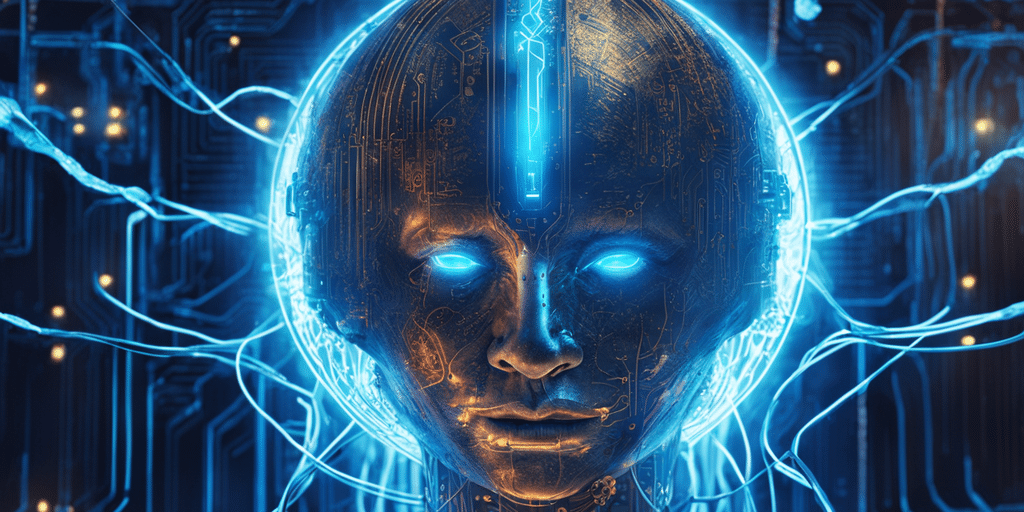
It was a corporate spy story that didn't even have a real human scriptwriter. OpenAI, the company that ignited the global AI obsession last year, found itself in the headlines after the sudden firing and reinstatement of CEO Sam Altman.
Even with Altman back where he started, a swirling cloud of questions remains, including what happened behind the scenes.
Some have described Chaos as an HBO-level “success” or a “Game of Thrones” war. Others speculated that it was because Altman was focusing on other companies like WorldCoin.
But the closest and most plausible theory says that it was fired because of one letter: Q.
Unnamed sources told Reuters that OpenAI CTO Mira Murati said the discovery, codenamed “Q Star” or “Q*,” was the impetus for the action against Altman, which killed board chairman Greg Brockman without involvement, and later resigned. In opposition to OpenAI.
What in the world is “Q*” and why should we care? It's all about the paths AI development can take from here.
Table of Contents
ToggleRevealing the secret of Q*
The puzzle Q* cited by OpenAI's CTO Mira Murati has sparked widespread speculation in the AI community. This term can refer to one of two different theories: Q-Learning or Q*algorithm from the Maryland Refutation Proofing Procedure System (MRPPS). Understanding the difference between the two is critical to understanding the potential impact of Q*.
Theory 1: Q-Learning
Q-Learning is a form of reinforcement learning, a method in which AI learns to make decisions through trial and error. In Q-Learning, an agent learns to make decisions by estimating the “quality” of action-state combinations.
The difference between this approach and OpenAI's current approach – Reinforcement Learning with Human Feedback or RLHF – is that it doesn't rely on human interaction and does everything by itself.
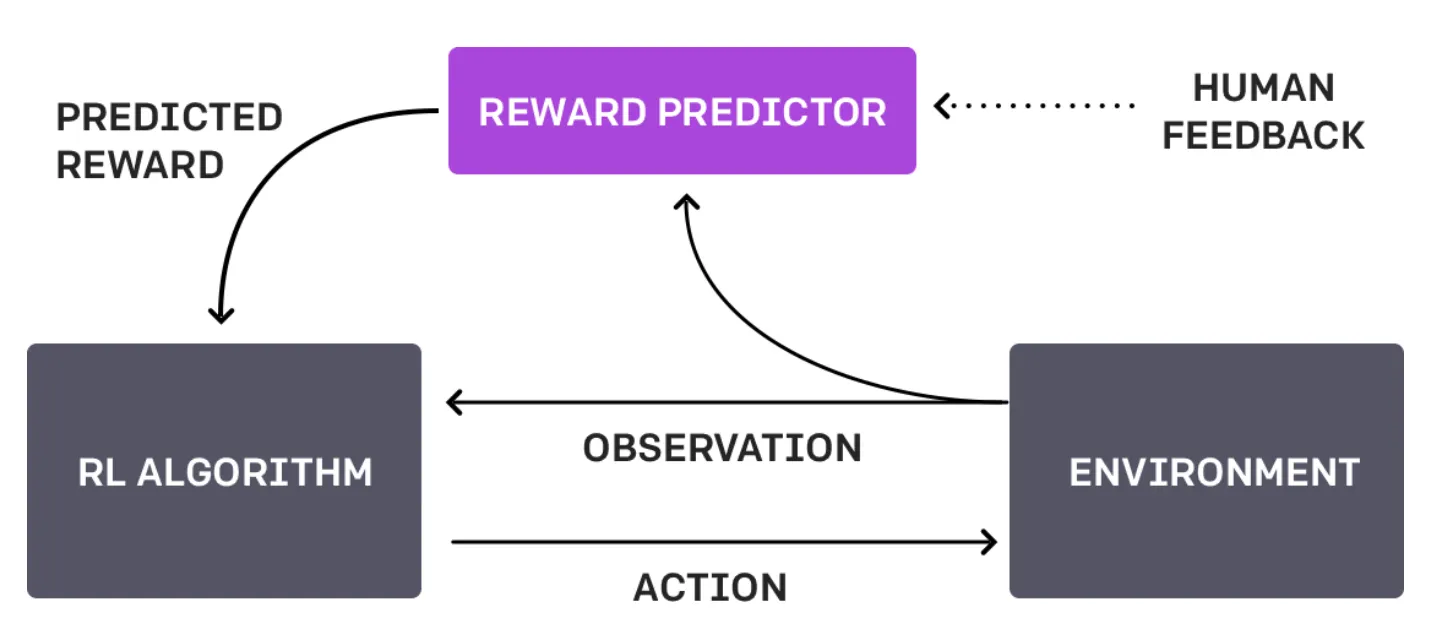
Imagine a robot moving through a maze. With Q-Learning, he learns to find the fastest way to the exit by trying different routes, receiving self-designed positive rewards as he approaches the exit, and receiving negative rewards as he reaches the end. Over time, through trial and error, the robot develops a strategy (“Q-table”), which tells it the best action from each position in the sequence. This process is autonomous based on the robot's interaction with its environment.
If the robot uses RLHF, instead of checking things out on its own, a human intervenes when the robot reaches an intersection and indicates whether the robot's choice is wise or not.
This feedback can be in the form of direct commands (“turn left”), suggestions (“try the path with more light”), or evaluations of the robot's choices (“good robot” or “bad robot”).
In Q-Learning, Q* represents the desired state in which the agent knows exactly the best action to take in each state to maximize its expected total reward over time. Mathematically, it satisfies the Bellman equation.

In May, OpenAI published a paper that “Trained a model to bring a new state-of-the-art approach to math problem solving by rewarding each correct reasoning step instead of simply rewarding the final answer.” If you use Q-Learning or a similar method to achieve this, that opens up a whole new set of problems and scenarios that ChatGPT can natively solve.
Theorem 2: Q* algorithm from MRPPS
The Q* algorithm is part of the Maryland Refusal Proof Procedure System (MRPPS). It is a sophisticated technique for theory proofing in AI, especially in question-answering systems.
“The Q∗ algorithm generates nodes in the search space, using semantic and syntactic information to guide the search. Hermeneutics allows paths to be crossed and fruitful paths to be explored,” the research paper reads.
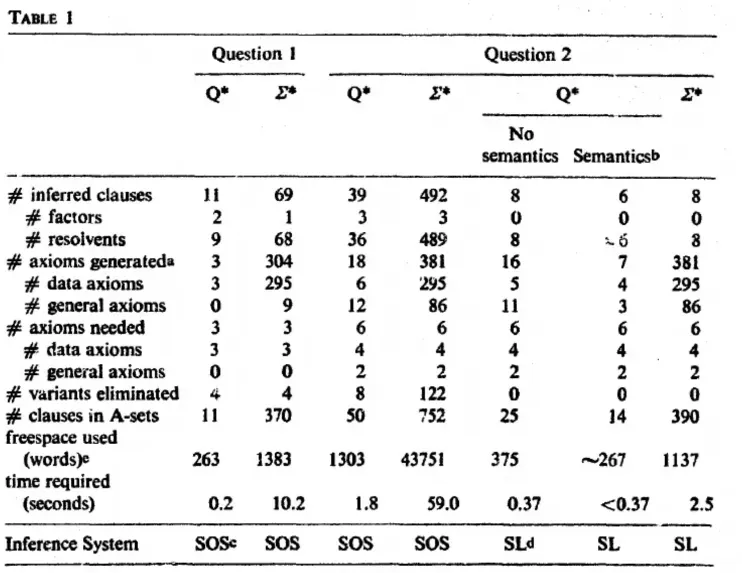
One way to explain the process is to consider Sherlock Holmes, a fictional detective trying to solve a complex case. It gathers clues (semantic information) and connects them logically (syntactic information) to reach a conclusion. The Q* algorithm works similarly in AI, combining semantic and syntactic information to explore complex problem-solving processes.
This indicates that OpenAI is one step closer to moving beyond real-world text queries and toward a real-world understanding model akin to a realistic JARVIS (for GenZers) or a bat computer (for Boomers).
So, while Q-learning is about teaching AI to learn through interactions with its environment, Q algorithm is about improving AI's deductive abilities. Understanding these differences is key to appreciating the potential implications of OpenAI's “Q.” Both have great potential in advancing AI, but their applications and implications differ significantly.
This is all just speculation, of course, as OpenAI hasn't explained the concept or even confirmed or denied the rumor that it actually exists, whatever it may be.
Possible implications of ‘Q'*
OpenAI's rumored ‘Q*' could have wide and varied effects. If it's an advanced form of Q-Learning, this could imply that AI has the ability to autonomously learn and adapt to complex environments, solving a whole new set of problems. Such progress could improve AI applications in areas such as autonomous vehicles, where split-second decision making based on constantly changing conditions is essential.
On the other hand, if ‘Q' corresponds to the Q algorithm from MRPPS, it could represent a major step forward in AI's deductive reasoning and problem-solving capabilities. This is especially useful in fields that require deep analytical thinking, such as legal analysis, complex data interpretation, and even medical diagnosis.
Regardless of its exact nature, ‘Q*' can represent a major advance in AI development, so the reality in OpenAI's survival argument is true. It could bring us closer to AI systems that are more intuitive, efficient and capable of tasks that currently require a high level of human intelligence. However, with such advances come questions and concerns about AI ethics, safety, and the ever-increasing impact of AI systems on our daily lives and society as a whole.
The good and bad of Q*
Potential benefits of Q* include:
Improved problem solving and efficiency: If Q* is an advanced Q-Learning or Q*algorithm, it could lead to AI systems that efficiently solve complex problems, benefiting sectors such as healthcare, finance and environmental management.
Better human-AI collaboration: AI with improved learning or deductive capabilities can augment human work, leading to more effective collaboration in research, innovation, and everyday tasks.
Advances in Automation: ‘Q*' leads to advanced automation technologies, which can improve productivity and create new industries and job opportunities.
Risks and Concerns:
Ethical and safety issues: As AI systems become more sophisticated, ensuring that they operate ethically and safely becomes increasingly challenging. There is a risk of unintended consequences, especially if AIA actions are not fully aligned with human values.
Privacy and Security: With more advanced AI, privacy and data security concerns are increasing. AI systems capable of deep understanding and interaction with data can be misused. So, imagine an AI that will call you when you cheat on your romantic partner because it knows that cheating is bad.
Economic Impacts: Increasing automation and AI capabilities could lead to job displacement in certain sectors, societal restructuring and new approaches to workforce development. If AI can do almost everything, why do they have human employees?
AI Misalignment: The risk that AI systems misalign goals or mechanisms with human needs or well-being, leading to harmful outcomes. Imagine a cleaning robot that is obsessed with cleanliness and throws away your important papers? Or does it eliminate the troublemakers altogether?
The legend of AGI
Where does OpenAI's rumored Q* stand amid Artificial General Intelligence (AGI) – AI Research's Search for the Saints?
AGI is the machine's ability to understand, learn, and perform tasks similar to human cognitive abilities. It is a type of AI that can generalize learning from one domain to another, demonstrating true adaptability and versatility.
While Q is an advanced form of Q-learning or related to the Q algorithm, it is important to understand that this is not the same as discovering AGI. While ‘Q*' may represent a big step forward in certain AI capabilities, AGI encompasses a wider range of skills and understandings.
Achieving AGI means developing an AI that can perform almost any intellectual task a human can – an elusive milestone.
A machine that achieves Q does not know its own existence and cannot yet go beyond the limits of pre-trained data and human crowdsourcing algorithms. So no, despite all the hype, “Q” is not yet anathema to our AI overlords. He's like a smart toaster who learned to butter his own bread.
As for AGI ushering in the end of civilization, we may be overestimating our importance on a cosmic scale. OpenAI's Q* may be a step closer to the AI of our dreams (or nightmares), but it's not an AGI that contemplates the meaning of life or its own silicon existence.
Remember, this is the parent who was watching the chatgpt attentively watching the toddler with a pointer – proud, but forever drawn to the wall of humanity. When “Q*” jumps, the AGI is another bound, and the human tower is safe for now.
Edited by Ryan Ozawa.